How to carry out ISO 42001 AI risk assessments

ISO/IEC 42001:2023 is the new kid on the block regarding compliance standards. Released at the end of 2023, it establishes guidelines for building an Artificial Intelligence Management System (AIMS). We reviewed the standard at a high level in a separate post, but we will delve into AI risk assessments in this one.
Clause 6.1.2 of the standard requires organizations to “define and establish an AI risk assessment process.†It offers a few additional requirements for how to do so, such as requiring these assessments to consider “potential consequences to the organization, individuals, and societies.†However, it doesn't offer detailed guidance.
A separate publication, ISO/IEC 23894:2023, specifically focuses on AI risk assessments, but even that leaves something to be desired regarding real-world applications. So in this blog, we'll offer some practical recommendations on how to go about AI risk assessments before the ISO 42001 certification process.
Think broadly, but don't boil the ocean
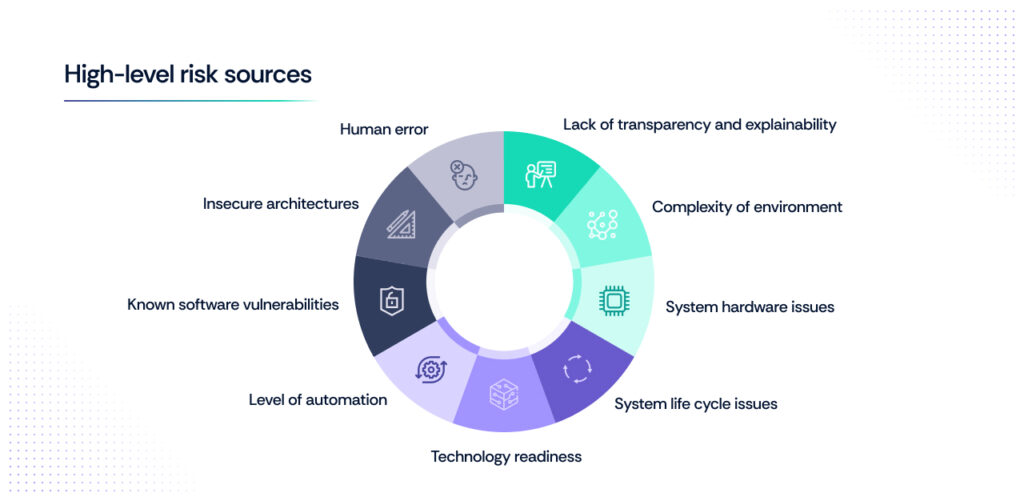
ISO 23894 provides a useful set of high-level risk sources that can help spur your risk management brainstorming process, including:
- Lack of transparency and explainability.
- Complexity of environment.
- System hardware issues.
- System life cycle issues.
- Technology readiness.
- Level of automation.
With that said, these aren't necessarily comprehensive. While ISO/IEC 27001:2022 focuses on information security and privacy, there is some overlap with ISO 42001. Thus, it would also make sense to consider familiar sources such as:
- Known software vulnerabilities.
- Insecure architectures.
- Human error.
In addition to the sources of risk, you'll need to identify assets and their value. These include various levels such as:
- Organizational, e.g., AI models and underlying data.
- Personal: e.g., private health information.
- Societal: e.g., the environment.
The key is not to develop an infinitely long catalog of risk sources and assets. Customize them to your company and its business operations for the greatest effectiveness.
1. Choose your approach
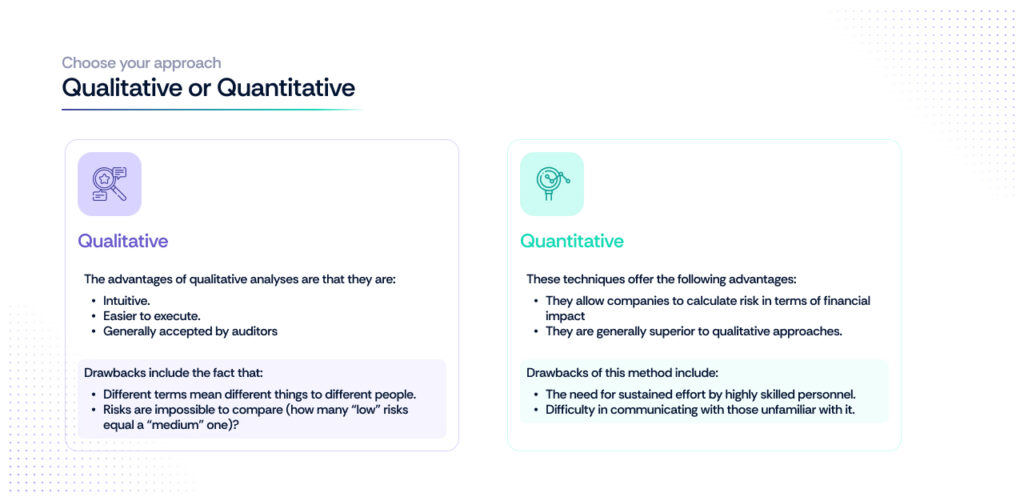
ISO 42001 doesn't prescribe a specific risk assessment approach. ISO 23894 merely states that “AI risks should be identified, quantified or qualitatively described and prioritized.†So companies can pursue two different methods:
Qualitative
Probably the most common approach is categorizing risks with terms such as “high†or “low.†Other standards, like the Common Vulnerability Scoring System (CVSS) create arbitrary 0-10 scales that are still qualitative (although the organization that publishes the standard is not entirely clear if CVSS is meant to describe risk or not).
The advantages of qualitative analyses are that they are:
- Intuitive.
- Easier to execute.
- Generally accepted by auditors.
Drawbacks include the fact that:
- Different terms mean different things to different people.
- Risks are impossible to compare (how many “low†risks equal a “medium†one)?
If your organization is just starting out, a qualitative approach might be the most appropriate one. But if you are at a more advanced level, pursuing a more definitive method would make sense.
Quantitative
The gold standard of risk assessment is quantitative. The Factor Analysis of Information Risk (FAIR) methodology is probably the most common method. AI-specific quantitative solutions have also emerged, like the Artificial Intelligence Risk Scoring System (AIRSS).
These techniques offer the following advantages:
- They allow companies to calculate risk in terms of financial impact
- They are generally superior to qualitative approaches.
Drawbacks of this method include:
- The need for sustained effort by highly skilled personnel.
- Difficulty in communicating with those unfamiliar with it.
2. Document impact

Once you have selected your method, the next step is evaluating the consequences of a given risk materializing. ISO 23894 requires breaking these down into business, individual, and societal impacts.
A. Business
At the business level, if you were evaluating the impact of model collapse on operations, you might depict impact in the following ways, depending on your approach:
- Qualitative: Model collapse would result in critical disruption to business operations.
- Quantitative: Single loss expectancy (SLE) from a model collapse event would be $1,000,000 due to the requirement to divert workers to complete automated tasks while we find a new AI system.
B. Individual
At a personal level, an AI risk assessment for unintended training on personal data could look like:
- Qualitative: There would be a high impact on personal privacy from unintended model training on personal information.
- Quantitative: Based on historical events, the unintended training on a person's social security number has an SLE of $9.2.
C. Societies
Finally, on the societal level, one could depict the consequences of AI-related power outage risk materializing in the following ways:
- Qualitative: There would be a moderate impact on society from AI-induced power outages.
- Quantitative: Because the United States suffers approximately $150 billion per year in economic damage from power outages, and non-weather related outages are 13% of the total ($19.5 billion), we assess a range of damage per event between $1 million and $100 million.
D. Spell it out for the auditor
Documenting your justifications is a key aspect of the exercise. Because predicting future damage from an AI-related event is so difficult, no one expects you to do it perfectly. What auditors will demand, however, is that you justify your estimates.
Additionally, for the purposes of ISO 42001, it is important to be explicit about why an outcome is bad. It is not enough to describe a potential consequence like “safety layer evasion†and assume this to be self-explanatory.
Auditors will expect you to document that this can lead to:
- Generation of illegal, harmful, or infringing content.
- Reputation damage for the organization.
- Reduced trust in the AI system.
3. Assess likelihood
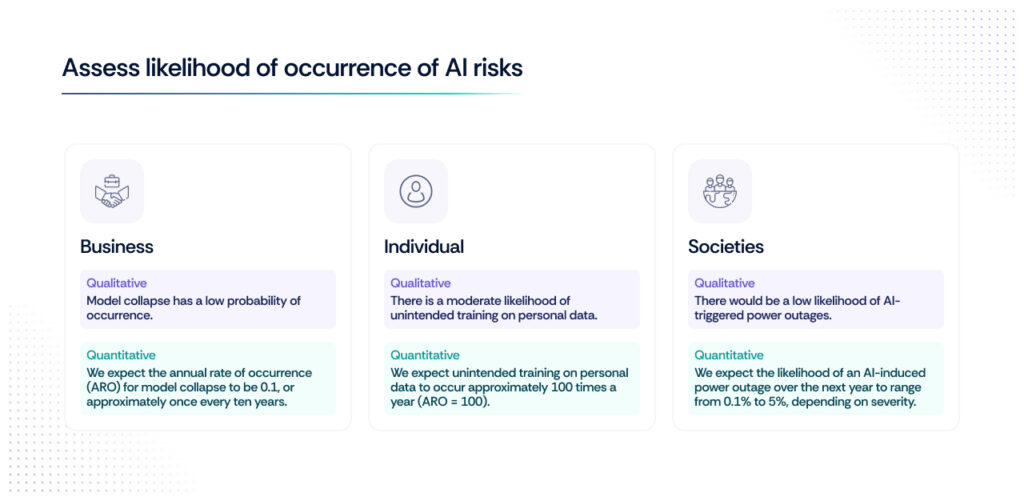
Once you are clear on the consequences of the relevant AI-related risks, it's time to assess their likelihood of occurring. Using the examples from the previous section, we might document the following:
Business
- Qualitative: Model collapse has a low probability of occurrence.
- Quantitative: We expect the annual rate of occurrence (ARO) for model collapse to be 0.1, or approximately once every ten years.
Individual
- Qualitative: There is a moderate likelihood of unintended training on personal data.
- Quantitative: We expect unintended training on personal data to occur approximately 100 times a year (ARO = 100).
Societies
- Qualitative: There would be a low likelihood of AI-triggered power outages.
- Quantitative: We expect the likelihood of an AI-induced power outage over the next year to range from 0.1% to 5%, depending on severity.
4. Calculate overall risk
This is where things get challenging for qualitative approaches. Quantitative methods shine in contrast.
The former would require some sort of heat map or similar matrix demonstrating that a high impact event with a low likelihood is equivalent to a moderate risk. The challenge here is that doing this can be imprecise and different people can reasonably disagree on the outcomes.
Quantitative approaches, however, are much cleaner and definitive. Using our three previous examples, we might arrive at the following outcomes:
Business
- Qualitative: Model collapse is a moderate risk.
- Quantitative: Using the AIRSS, the annual loss expectancy (ALE) of model collapse is $100,000.
Individual
- Qualitative: Unintended training on personal data is a moderate risk.
- Quantitative: Using the AIRSS, the ALE of unintended training on personal data risk is $920.
Societies
- Qualitative: AI-triggered power outages represent a low risk.
- Quantitative: Using the FAIR method, we developed a loss exceedance curve (LEC) depicting the risk of AI-induced power outages over the next year. At the low end, we expect the likelihood of a $1 million outage occurring to be 5%. At the high end, we expect the likelihood of a $100 million outage occurring to be 0.1%.
Conclusion
Risk assessments, including those for AI, are by no means a science. The “art†form comes into play when scoping the processing, selecting which method(s) to use, and deciding how to represent impact, likelihood, and overall risk rating.
ISO 42001 and 23894 provide some guidelines, but they are far from comprehensive references for AI risk assessment. Companies seeking certification under the former standard will generally have a lot of work to do to get ready for an external audit.
Are you considering ISO 42001 certification and need help building out your risk analyses? The good news is that Scrut Automation's smartGRC platform can help you do just that. Book a demo to see how we can streamline your compliance and security program.
Ready to see what security-first GRC really looks like?
Ready to see what security-first GRC really looks like?
Ready to see what security-first GRC really looks like?
See what a real security- first GRC platform looks like
Ready to see what security-first GRC really looks like?
Focus on the traveler experience. We’ll handle the regulations.
Get Scrut. Achieve and maintain compliance without the busywork.
Choose risk-first compliance that’s always on, built for you, and never in your way.
Ready to see what security-first GRC
One platform, every framework. No more duplicate work.
You can’t manage user access if you’re always playing catch-up.
Explore the future of enterprise GRC
Tired of chasing vendors for risk assessments?
Join the thousands of companies automating their compliance with Scrut.
The right partner makes all the difference. Let’s grow together.
Make your business easy to trust, put security transparency front and center.
Risk-first security starts with risk-first visibility.
Secure your team from the inside out.
Don't settle for slow, expensive compliance. Get Scrut instead.
Risk-first compliance for forward-thinking teams.
Audits without the back-and-forth. Just seamless collaboration.
Scale fast. Stay compliant. Automate the rest.
Compliance? Done and dusted, in half the time.
Get ahead of GDPR compliance before it becomes a problem.
Outgrowing table-stakes compliance? Create custom frameworks with ease.
Navigate SOC 2 compliance, minus the stress.
PCI DSS compliance, minus the panic.
Take the wheel of your HIPAA certification journey today.
We’ve got what you need to fast-track your ISO 27001 certification.
Make your NIST AI RMF journey as smooth as possible.
Your GRC team, multiplied and AI-backed.
Modern compliance for the evolving education landscape.
Ready to simplify healthcare compliance?
Don’t let compliance turn into a bottleneck in your SaaS growth.
Find the right compliance frameworks for your business in minutes
Ready to see what security-first GRC really looks like?
Real-time visibility into every asset
Ready to simplify fintech compliance?
The Scrut Platform helps you move fast, stay compliant, and build securely from the start.
Scrut helps you set up a security program that scales with your business and stands up to audits. Without last-minute chaos.
Scrut helps you streamline audits, close deals faster, and stay ahead of risk without slowing down your team. Because trust shouldn’t take months to earn.
Scrut helps you set up a security program that scales with your business and stands up to audits. Without last-minute chaos.
Tag, classify, and monitor assets in real time—without the manual overhead.
Whether you're entering new markets or launching new products, Scrut helps you stay compliant without slowing down.
Scrut pulls compliance data straight from the tools you already use—so you don’t have to dig for evidence, chase approvals, or manually track controls.
Less manual work, more customizability. The Scrut Platform gives you everything you need to align your compliance to your business’s priorities.
With Scrut, you’re not just adding a tool to your offering—you’re adding a competitive edge. Join our Partner Network and help your clients streamline their GRC program.
Gaining trust is your first step to growing and cracking better deals. The Scrut Platform comes pre-built with all the tools you need to showcase a firm security posture and build confidence.
Don’t settle for rigid systems—Scrut ensures your risk management strategy is as flexible as your business needs.
Start building a security-first culture. Save your operations from improper training and a lack of compliance awareness.
Scrut fast-tracks compliance so you can focus on scaling, not scrambling. Automate compliance tasks and accelerate enterprise deals—without the grind.
Automate assessments, track compliance, and get full visibility into third-party risk—all in one place.
Scrut automates compliance tasks, supports proactive risk management, and saves you time, so you can focus on growing your business. Start building trust with customers and scaling confidently.
Leave legacy GRC behind. Meet the AI-powered platform built for teams managing risk and compliance in real time.
Give auditors direct access, keep track of every request, and manage audits effortlessly—all in one place.
Scrut ensures access permissions are correct, up-to-date, and fully compliant.
Whether you need fast results or a fully tailored program mapped to your risks and needs, Scrut delivers exactly what you need, when you need it. Ready to start?
Scrut unifies compliance across all your frameworks, so you can stop juggling systems and start scaling securely.
Manually managing your compliance processes and audits can get inefficient and overwhelming. Scrut automates these outdated, manual processes and eliminates your last-minute worries.
Access automated compliance, real-time risk tracking, and expert-backed support—all in one platform. Get started with Scrut!
Less manual work, more customizability. The Scrut Platform gives you everything you need to align your compliance to your business’s priorities.
The Scrut Platform helps you move fast, stay compliant, and build securely from the start.
Earn trust and back it up with solid evidence. Scrut takes you through the SOC 2 compliance journey step-by-step, navigating every complexity you face.
Manage your PCI DSS compliance with real-time monitoring and effortless automation. Get started with Scrut today!
Securing your PHI shouldn’t be a constant hassle. Scrut automates your workflows—from risk assessments to monitoring—so you can put your compliance worries on the back burner.
Automate security controls, simplify audits, and keep your ISMS aligned with the latest standards. Get started with Scrut!
Tackle potential AI risks with NIST AI RMF-compliant controls and get expert support every step of the way.
Offload the grunt compliance work to us. Execute manual, draining GRC tasks with the reliable AI-powered Scrut Teammates without switching contexts or bottlenecks.
Whether you're managing student data, partnering with educational institute, or expanding to new geographies—Scrut gives you the tools to stay compliant, manage risk, and build trust at every step.
Scaling healthcare doesn’t have to come at the cost of security. Scrut keeps your organization compliant, audit-ready, and protected—no matter how fast you grow.
Scrut automates the hard parts of compliance and security so you can move fast and stay ahead of risks from day one.
The Scrut Platform helps you move fast, stay compliant, and build securely from the start.
Growth in fintech comes with heavy scrutiny. Scrut helps you stay compliant, audit-ready, and secure—without slowing down your momentum.

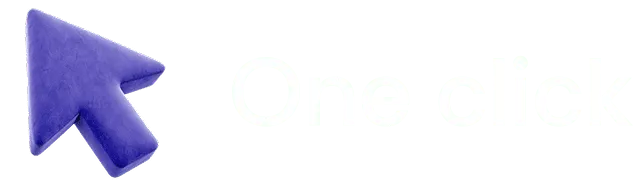
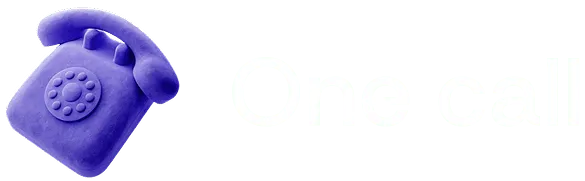
