As Artificial Intelligence (AI) technologies become more widespread, managing risks such as bias, security vulnerabilities, and unpredictability is increasingly important.
The NIST AI Risk Management Framework (RMF) 1.0 helps organizations manage these risks throughout the AI lifecycle. By following the NIST AI RMF 1.0, businesses can balance innovation with responsible AI development, ensuring reliable and ethical systems.
In this blog, we’ll explore the NIST AI Risk Management Framework 1.0, its purpose, key components, implementation, and associated challenges.
What is the NIST AI Risk Management Framework?
According to NIST’s official documentation, it “developed the voluntary NIST AI RMF to help individuals, organizations, and society manage AI’s many risks and promote trustworthy development and responsible use of AI systems. NIST was directed to prepare the Framework by the National Artificial Intelligence Initiative Act of 2020 (P.L.116-283).”
Developed in response to a Congressional mandate and through extensive collaboration with both public and private sectors, it provides flexible guidelines to keep pace with rapid AI advancements.
Directed by the National Artificial Intelligence Initiative Act of 2020, the voluntary framework offers a structured approach to identifying, assessing, and mitigating AI-related risks while promoting trustworthy AI. It enhances fairness, safety, privacy, and security, making it applicable to organizations across all industries, including those in regulated sectors and those handling sensitive data.
By standardizing AI risk management, NIST AI RMF 1.0 helps organizations make more informed decisions, minimize potential harm, and build public trust in AI technologies.
Audience of AI RMF
The NIST AI RMF applies to stakeholders across the AI lifecycle, ensuring diverse teams manage both technical and societal AI risks. Key actors span several lifecycle areas: application context, data, AI models, and task outputs.
The audience of AI RMF includes:
- AI experts specializing in Testing, Evaluation, Verification, and Validation (TEVV) are crucial, offering insights into the technical and ethical dimensions of AI systems.
- Additionally, trade associations, advocacy groups, and impacted communities contribute to discussions on AI risk management by addressing societal, environmental, and ethical concerns, offering guidance, and weighing trade-offs between values like civil liberties, equity, and environmental impact.
Ultimately, effective AI risk management requires a collective effort, where diverse teams collaborate to share knowledge, identify risks, and ensure AI technologies align with broader societal goals.
What are the core functions of the NIST AI RMF?
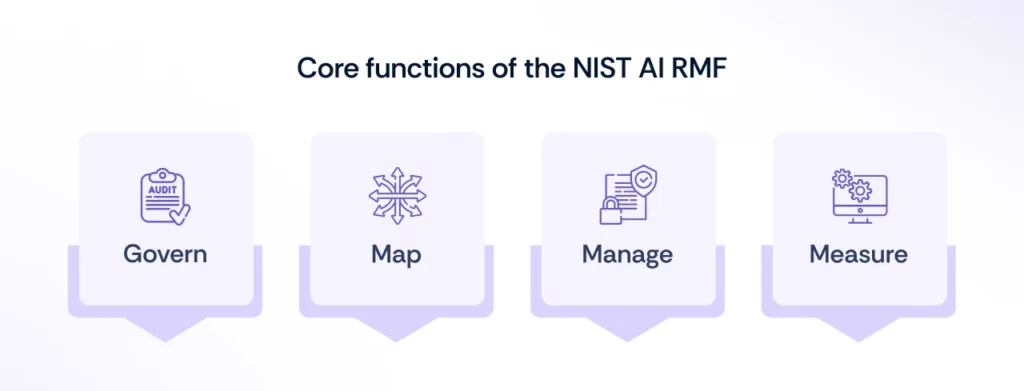
The NIST AI RMF emphasizes essential practices for building trustworthy and responsible AI systems, with a focus on governance, risk assessment, and risk mitigation strategies.
The framework outlines four core functions necessary for its successful implementation. These functions help organizations manage AI risks holistically, ensuring ethical, societal, operational, and security considerations are addressed.
1. Govern
The GOVERN function creates a culture of AI risk management by integrating policies, processes, and accountability across the organization. It ensures risks are identified, assessed, and managed through clear documentation, ethical considerations, legal compliance, and transparency. This function aligns AI development with organizational values, linking risk management to strategic goals and covering the full AI lifecycle, including third-party risks.
Key elements of GOVERN include:
- Risk management: Establishes policies and procedures to map, measure, and manage AI risks in alignment with organizational risk tolerance and external expectations.
- Accountability: Defines clear roles and responsibilities, ensuring that personnel are trained and empowered to manage AI risks.
- Interdisciplinary perspectives: Encourages input from diverse stakeholders, including legal, ethical, and technical experts, to enhance AI governance and decision-making.
- External engagement: Collects and integrates feedback from AI stakeholders and addresses risks related to third-party systems and supply chain dependencies.
This cross-functional approach ensures that AI risk management aligns with organizational priorities and adapts to evolving needs.
2. Map
The MAP function sets the context for identifying and understanding risks throughout the AI lifecycle, anticipating potential impacts at each stage. It clarifies risks related to the AI system’s usage, including the roles of actors and external stakeholders, as well as interdependencies and varying control levels.
Key elements of the MAP function include:
- Contextual understanding: Defines the AI system’s purpose, benefits, risks, and compliance with laws, norms, and user expectations.
- System categorization: Documents tasks, methods, knowledge limits, and scientific integrity.
- Capabilities and benefits: Assesses expected benefits, costs (monetary and non-monetary), performance, trustworthiness, and human oversight.
- Risk mapping: Identifies and documents risks across all AI lifecycle stages, including those arising from third-party software and external data sources.
- Impact assessment: Evaluates the AI system’s potential effects on individuals, communities, organizations, and society, considering ethical and societal implications.
Completing the MAP function gives organizations the knowledge needed to decide whether to move forward with the AI system. This information guides the next steps in the MEASURE and MANAGE functions and ensures that AI risk management stays aligned with changing contexts and potential impacts.
3. Measure
The MEASURE function uses quantitative, qualitative, or mixed-method tools to assess, monitor, and continuously evaluate AI risks and their impacts. This function builds upon the risks identified in the MAP function and informs the MANAGE function, enabling organizations to assess the trustworthiness, fairness, robustness, and overall performance of AI systems.
Key elements of the MEASURE function include:
- Risk measurement and monitoring: Applies appropriate metrics to assess AI risks, trustworthiness, and potential unintended consequences.
- AI evaluation: Assesses AI systems for key characteristics such as fairness, transparency, explainability, robustness, safety, and privacy.
- Testing and validation: Involves rigorous testing using scientific, ethical, and regulatory standards, documenting results focused on reliability, safety, and security.
- Emergent risk tracking: Implements mechanisms to identify and track unforeseen risks over time, leveraging stakeholder feedback.
- Feedback and continuous improvement: Evaluates measurement effectiveness by gathering input from experts and stakeholders, ensuring AI systems remain aligned with evolving objectives.
By completing the MEASURE function, organizations create objective, repeatable, and transparent processes for evaluating AI system trustworthiness, allowing them to track and mitigate risks effectively. The results feed into the MANAGE function, supporting proactive risk response and adaptation.
4. Manage
The MANAGE function comes last in the process and focuses on allocating resources for AI risk management based on assessments from the MAP and MEASURE functions. It involves developing strategies to mitigate, respond to, and recover from AI-related risks, ensuring regular monitoring and continuous improvement of AI systems.
Key elements of the MANAGE function include:
- Risk prioritization and response: Dynamically prioritizes risks identified through MAP and MEASURE based on impact, likelihood, and resources. Plans must remain adaptable, with options like mitigation, transfer, or acceptance.
- Maximizing AI benefits and minimizing negative impacts: Develops strategies to enhance AI benefits, evaluate resource needs, and sustain AI system value while planning for unknown risks.
- Third-party risk management: Regularly monitors and manages risks from third-party AI components, such as pre-trained models or external data, ensuring they align with organizational risk strategies.
- Risk treatment and monitoring: Creates ongoing post-deployment monitoring plans to identify and respond to AI failures, stakeholder concerns, and emerging risks.
- Communication and documentation: Establishes proactive communication plans to inform relevant AI actors and affected communities about incidents and system performance, ensuring transparency and accountability.
The MANAGE function ensures that AI systems are actively monitored, risks are continuously reassessed, and response strategies evolve over time to maintain AI system integrity and alignment with organizational goals.
Together, these four functions create a comprehensive framework for AI risk management, addressing ethical, societal, operational, and security concerns. By following the NIST AI RMF, organizations can enhance AI governance, build trustworthy AI systems, and effectively respond to evolving risks.
What are the risks of AI Management?
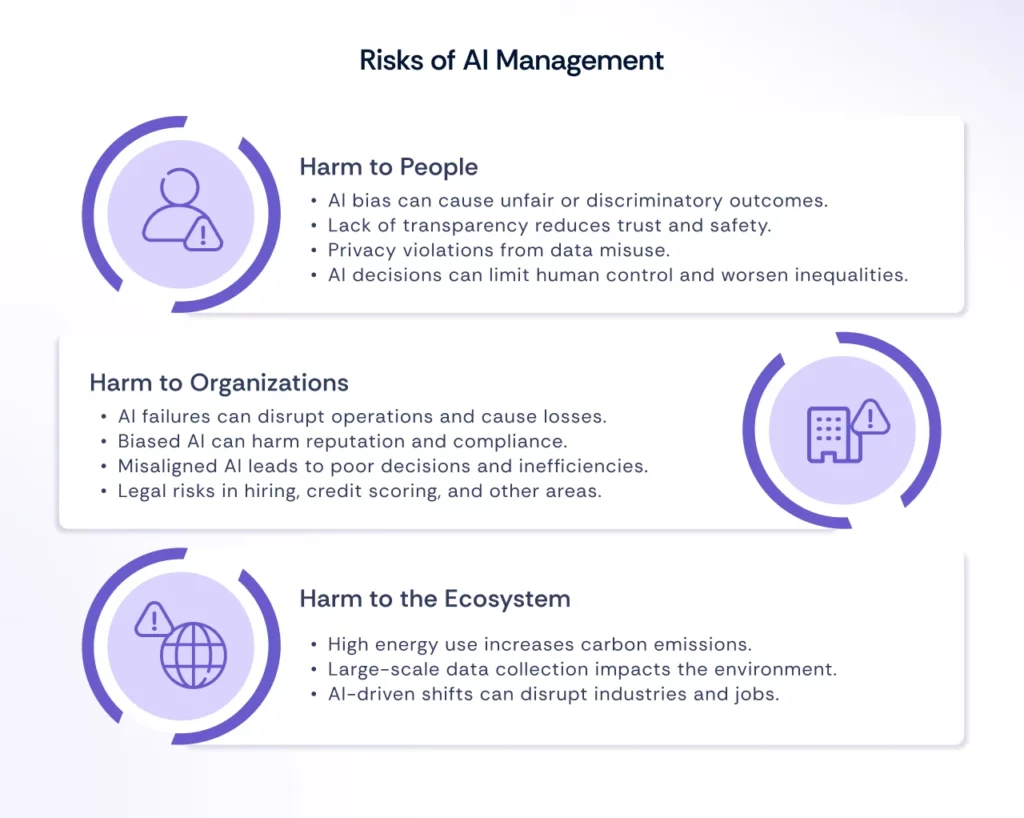
As AI technology continues to evolve, it brings both opportunities and challenges, with potential risks that can impact individuals, organizations, and ecosystems in various ways.
1. Harm to people
AI systems can significantly harm individuals, groups, communities, and society, especially when they are biased, lack transparency, or operate unpredictably.
- Bias in AI algorithms can lead to unfair treatment or discriminatory outcomes, affecting marginalized groups.
- Privacy violations can occur when AI systems misuse or mishandle personal data. They can harm a person’s safety.
- AI-driven decisions can undermine human agency and exacerbate inequalities.
2. Harm to organizations
For organizations, AI poses operational and reputational risks.
- AI systems can malfunction, harming business operations, and leading to financial losses, security breaches, or compliance failures, which can lead to a loss of reputation.
- Misalignment between AI outputs and business goals can result in poor decision-making or operational inefficiencies.
- If an AI system is used to make critical decisions, like hiring or credit scoring, it can also introduce legal and ethical challenges if it is found to be biased or discriminatory.
3. Harm to ecosystem
AI systems, particularly those using large-scale data collection, can have unintended environmental impacts.
- The energy consumption required for training and running AI models can contribute to carbon emissions and environmental degradation.
- AI’s influence on global supply chains and production systems can disrupt ecosystems, leading to economic imbalances or even the displacement of certain industries or labor markets.
By managing these risks, organizations can foster the development of ethical, fair, and transparent AI systems that mitigate potential harms.
What are the AI RMF Profiles?
AI RMF profiles are tailored implementations of the NIST AI Risk Management Framework (AI RMF), aligning its core functions with specific applications, sectors, or risk management objectives. These profiles take into account sector-specific risks and best practices rather than just risk tolerance and resources. For instance, an organization might develop an AI RMF profile focused on hiring practices or fair housing compliance to address sector-specific risks. They provide insights into managing AI risks at various stages of the AI lifecycle or within specific sectors, technologies, or end-use contexts.
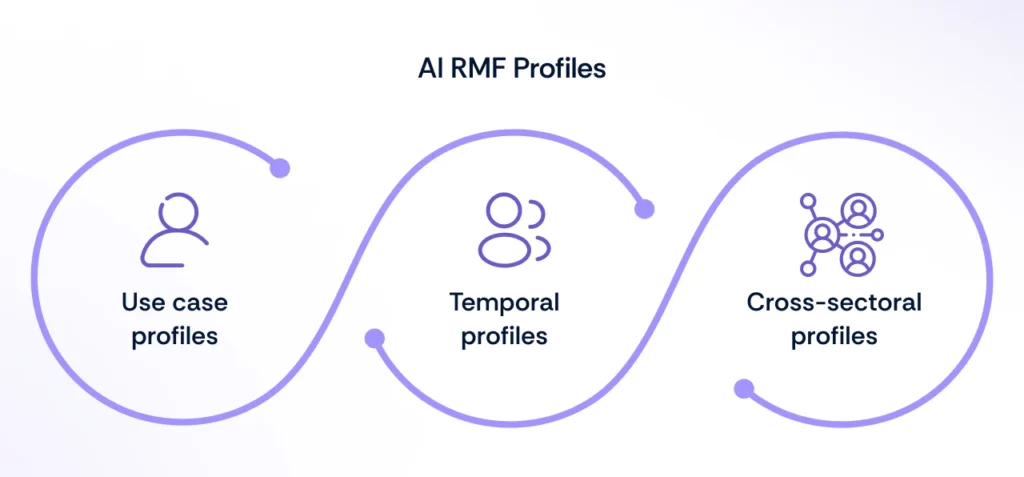
1. Use case profiles
AI RMF use-case profiles adapt the framework’s functions to specific settings or applications based on user requirements, risk tolerance, and resources. Examples include profiles for hiring or fair housing.
These profiles help organizations manage AI risks in alignment with their goals, legal requirements, and best practices while considering risk priorities at different stages of the AI lifecycle or within specific sectors.
2. Temporal profiles
AI RMF temporal profiles describe the current and target states of AI risk management activities. A Current Profile reflects the organization’s existing AI governance practices, risk exposure, and compliance posture, while a Target Profile represents the desired outcomes for AI risk management. By comparing the Current and Target Profiles, organizations can identify gaps in AI risk management and develop structured improvement plans. This process supports prioritizing mitigation efforts based on user needs, resources, and risk management objectives.
3. Cross-sectoral profiles
Cross-sectoral profiles address risks shared across various sectors or use cases, such as those associated with large language models, cloud services, or procurement. These profiles offer guidance on governing, mapping, measuring, and managing risks that span multiple sectors. The framework does not prescribe specific profile templates, allowing for flexible implementation based on sector-specific needs.
Challenges for AI Risk Management
The following challenges should be considered when managing risks to ensure AI trustworthiness.
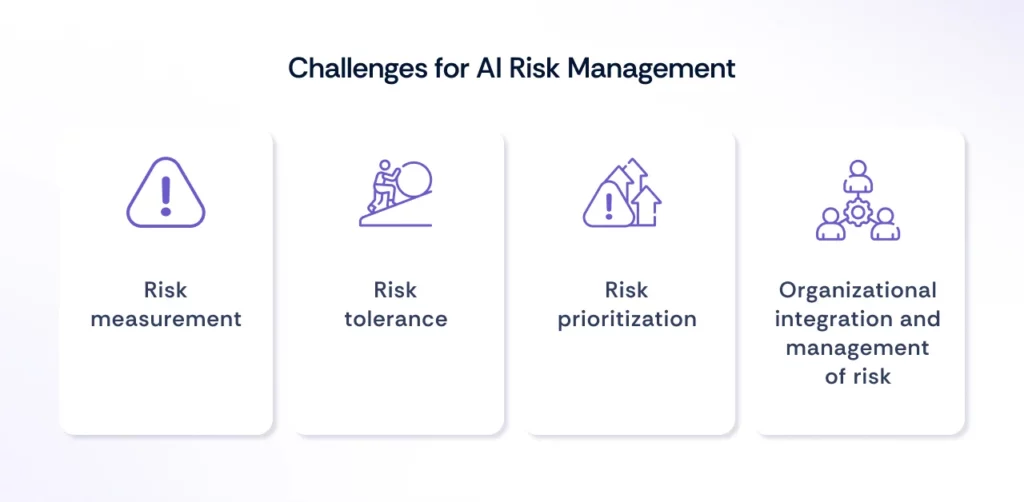
1. Risk measurement
AI risks that are not well-defined or understood are difficult to measure, whether quantitatively or qualitatively. While the inability to measure these risks doesn’t imply high or low risk, several challenges exist:
- Third-party risks: Third-party data, software, or hardware can accelerate development but complicate risk measurement. Mismatched risk metrics between developers and deployers, along with a lack of transparency, further complicate the process.
- Tracking emergent risks: Identifying and tracking new risks is crucial to improve risk management efforts. AI system impact assessments can help understand potential harms within specific contexts.
- Reliable metrics: A lack of consensus on standardized, verifiable risk measurement methods across various AI use cases is a challenge. Simplified or inadequate metrics may overlook critical nuances or fail to account for differences in affected groups.
- AI lifecycle risks: Risk measurement can vary throughout the AI lifecycle. Early-stage measurements may not capture latent risks that emerge later, and different AI actors may perceive risk differently.
- Real-world risks: Risks measured in controlled environments may differ from those observed in operational settings, adding complexity to real-world risk assessment.
- Inscrutability: Opaque AI systems, limited interpretability, or lack of transparency can hinder effective risk measurement.
- Human baseline: AI systems replacing or augmenting human tasks need baseline metrics for comparison, which is difficult to establish due to the unique ways AI performs tasks.
2. Risk tolerance
The AI RMF helps prioritize risks but does not define risk tolerance, which depends on an organization’s readiness to accept risk in pursuit of its goals.
Risk tolerance is influenced by legal, regulatory, and contextual factors, and it can vary based on policies, industry norms, and specific use cases. It may also evolve over time as AI systems and societal norms change.
Due to their priorities and resources, different organizations have different risk tolerances. As knowledge grows, businesses, governments, and other sectors will continue refining harm/cost-benefit tradeoffs, though challenges in defining AI risk tolerance may still limit its application in some contexts.
3. Risk prioritization
Eliminating all negative risks is often unrealistic, as not all incidents can be prevented. Overestimating risks can lead to inefficient resource allocation, wasting valuable assets. A strong risk management culture helps organizations understand that not all AI risks are the same, allowing for more purposeful resource distribution. Prioritization should be based on the assessed risk level and potential impact of each AI system.
When applying the AI RMF, higher-risk AI systems should receive the most urgent attention, with thorough risk management processes. If an AI system poses unacceptable risks, development and deployment should pause until risks can be addressed. Lower-risk AI systems may warrant less immediate prioritization, but regular reassessments remain essential, especially for AI systems with indirect impacts on humans.
Residual risks—those remaining after risk mitigation—can still affect users, and it’s important to document these risks so users are aware of potential impacts.
4. Organizational integration and management of risk
AI risks should be managed as part of broader enterprise risk strategies, as different actors in the AI lifecycle have varying responsibilities. For example, developers may lack insight into how the AI system is ultimately used. Integrating AI risk management with other critical risks like cybersecurity and privacy leads to more efficient, holistic outcomes.
The AI RMF can complement other frameworks for managing AI and broader enterprise risks. Common risks, such as privacy concerns, energy consumption, and security issues, overlap with those in other software development areas. Organizations should also establish clear accountability, roles, and incentive structures, with a senior-level commitment to effective risk management. This may require cultural shifts, particularly in smaller organizations.
What are the characteristics of trustworthy AI risk management?
To reduce risks, AI systems must meet several key criteria valued by stakeholders. This framework outlines essential characteristics of trustworthy AI: validity, safety, security, resilience, accountability, transparency, explainability, privacy, and fairness, with a focus on managing harmful bias.
These characteristics must be balanced according to the system’s specific use. While all attributes are socio-technical, accountability and transparency also relate to internal processes and external factors. Neglecting any of these can increase the risk of negative outcomes.
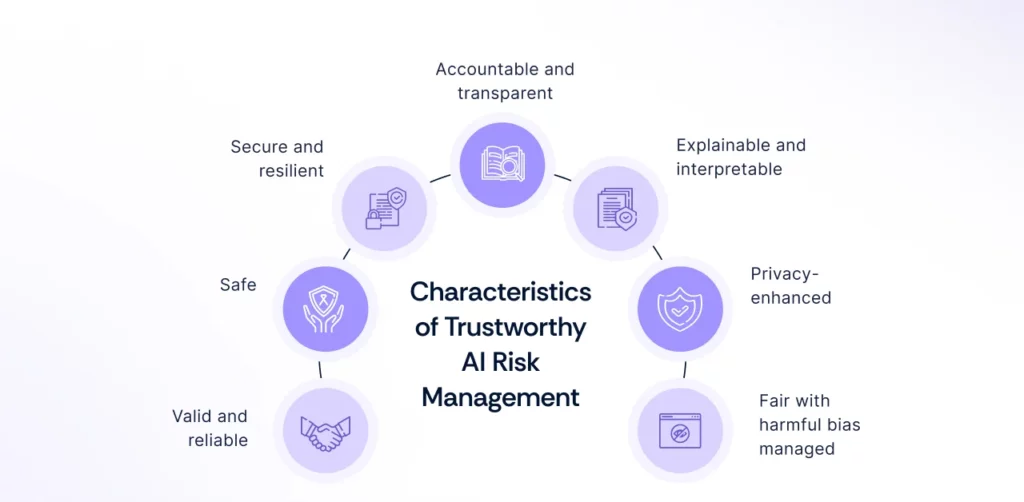
1. Valid and reliable
Validation ensures that an AI system fulfills its intended purpose with objective evidence, as outlined in ISO 9000:2015 for quality management. For AI-specific validation, ISO/IEC 23894:2023 provides a more tailored framework within the context of AI risk management. If AI systems are inaccurate or unreliable, they increase risks and reduce trust.
Reliability, defined as the system’s ability to perform without failure over time (ISO/IEC TS 5723:2022), ensures AI systems operate correctly throughout their lifecycle.
Accuracy measures the closeness of results to true values, while robustness ensures AI performs well across varied conditions. Balancing accuracy and robustness is key to AI trustworthiness, and these systems must be tested under realistic conditions. Ongoing monitoring and testing help ensure systems remain reliable and minimize harm, with human intervention necessary if errors are undetected or uncorrectable by the system itself.
2. Safe
AI systems must be designed to protect human life, health, property, and the environment. While ISO/IEC TS 5723:2022 offers guidance on AI system safety, additional standards such as ISO 21448 (SOTIF) and ISO/IEC 23894:2023 provide further considerations for managing AI-related risks.
Ensuring safety involves responsible design, clear guidelines for users, informed decision-making, and risk documentation based on real incidents. Safety risks vary by context, with high-risk scenarios (e.g., potential injury or death) requiring urgent prioritization and robust risk management.
Proactive safety measures should be integrated early in the design, along with simulations, real-time monitoring, and the ability for human intervention if systems deviate from expected behavior. Drawing on safety standards from industries like transportation and healthcare can further strengthen AI safety practices.
3. Secure and resilient
AI systems and their ecosystems are considered resilient if they can adapt to unexpected events or changes, maintain their functionality, and implement controlled fallback mechanisms when necessary (Adapted from ISO/IEC TS 5723:2022).
Common security risks include adversarial attacks, data poisoning, and the theft of models, training data, or intellectual property via AI system endpoints. Secure AI systems maintain confidentiality, integrity, and availability, using mechanisms to prevent unauthorized access and usage. Frameworks like NIST Cybersecurity and Risk Management provide relevant guidelines.
While security involves protecting, responding to, and recovering from attacks, resilience specifically refers to the ability to return to normal function after disruptions. Both concepts are interconnected but address different aspects of maintaining AI system stability and integrity.
4. Accountable and transparent
Trustworthy AI relies on accountability, which in turn depends on transparency. Transparency means providing relevant information about an AI system, its operations, and outputs to users or AI actors, tailored to their role and understanding. This can include details on design decisions, training data, model structure, intended use, and deployment decisions.
Transparency is essential for addressing issues like incorrect AI outputs and ensures accountability for AI outcomes. While transparency alone doesn’t guarantee accuracy, security, or fairness, it’s crucial for understanding and managing AI system behavior.
Practices to enhance transparency should be balanced with the need to protect proprietary information and consider cultural, legal, and sector-specific contexts, particularly when severe consequences are involved.
5. Explainable and interpretable
Explainability refers to understanding how an AI system works, while interpretability focuses on the meaning of its outputs. Both characteristics help users and operators better understand AI functionality, fostering trustworthiness. By enhancing explainability and interpretability, AI systems become easier to monitor, debug, and audit. These systems allow users to comprehend the reasons behind AI decisions, reducing risks and ensuring systems align with intended purposes. Transparency, explainability, and interpretability complement each other by answering the “what,” “how,” and “why” of AI system behavior.
6. Privacy-enhanced
Privacy in AI safeguards individual autonomy and identity by controlling data usage and preventing intrusion. Privacy risks may affect security, bias, and transparency, and often involve trade-offs.
AI systems should be designed with privacy values like anonymity and confidentiality in mind, using techniques like de-identification and aggregation. Privacy-enhancing technologies (PETs) can support these goals but may come at the cost of accuracy, which could impact fairness and other values.
7. Fair with harmful bias managed
Fairness in AI addresses equality and equity by managing harmful bias and discrimination. AI systems should recognize and mitigate various forms of bias—systemic, computational/statistical, and human-cognitive—that can arise unintentionally.
While mitigating bias is important, it doesn’t guarantee fairness in all cases. Bias can perpetuate or amplify harm, particularly in decisions about people’s lives, and it often ties into transparency and fairness within society. Recognizing different biases across the AI lifecycle is crucial for minimizing their impact and promoting fairness.
How to implement NIST AI RMF certification?
Implementing NIST AI RMF Certification involves a strategic approach that enables organizations to align their AI systems with standardized risk management practices. The process requires a combination of policies, controls, and automated processes to ensure that AI systems are secure, fair, and accountable.
The ultimate goal is to map AI systems to the NIST AI RMF framework and establish a robust program for continuous assessment and monitoring. This enables businesses to manage risks and comply with industry standards, fostering trust and transparency in their AI systems.
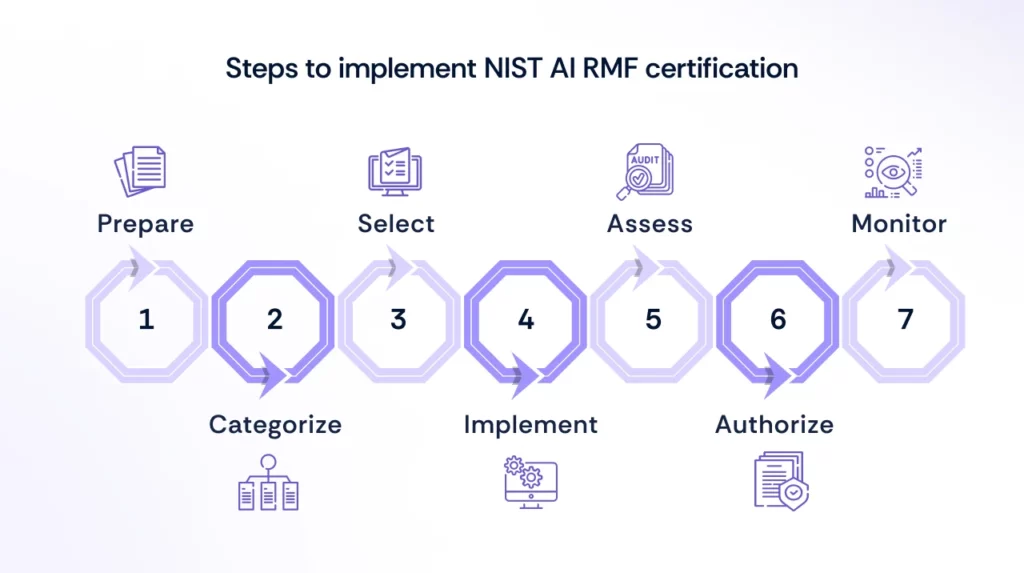
Steps to implement NIST AI RMF certification:
1. Prepare – Develop comprehensive policies and procedures that align with the NIST AI RMF framework to establish a strong foundation for risk management.
2. Categorize – Map AI systems to the framework, categorizing them based on their complexity, risk potential, and operational impact.
3. Select—Select risk management strategies and controls appropriate for the specific AI systems and use cases, ensuring compliance with NIST AI RMF guidelines.
4. Implement – Implement the selected policies, controls, and automated monitoring systems to ensure effective risk management across all AI systems.
5. Assess – Regularly assess the effectiveness of risk management strategies, ensuring that all controls are functioning properly and mitigating identified risks.
6. Authorize – Obtain authorization for AI systems, confirming that all necessary risk management practices are in place and compliant with NIST AI RMF.
7. Monitor – Continuously monitor AI systems to ensure ongoing compliance with NIST AI RMF standards and mitigate emerging risks effectively.
By following these steps, organizations can successfully implement NIST AI RMF Certification, ensuring that their AI systems are secure, compliant, and aligned with industry standards.
Also Read: NIST Compliance Software to Simplify Risk Management
Is there any AI RMF playbook to follow?
Yes, there is an AI RMF Playbook, which provides a structured approach for implementing the NIST AI RMF framework. It helps organizations manage AI risks by offering step-by-step guidance on categorizing, assessing, and monitoring risks. The playbook ensures AI systems align with best practices for transparency, ethics, and security. Following it fosters trust and mitigates operational and ethical risks in AI.
Do you need to do an AI risk assessment?
Yes, AI risk assessments are essential to identify and mitigate potential threats related to AI systems, such as bias, security vulnerabilities, and ethical concerns. Conducting these assessments helps ensure that AI models operate safely, transparently, and in compliance with regulations. It also supports organizations in building trust with stakeholders and maintaining accountability. Without regular risk assessments, AI systems may introduce unforeseen risks that could harm the organization or its users.
What are the pros and cons of implementing the NIST AI risk management framework?
Pros of implementing NIST AI RMF:
- Provides a structured approach to assess and mitigate AI risks.
- Enhances trust and transparency, building confidence in AI systems.
- Supports regulatory compliance, reducing legal risks.
- Allows tailored risk management through AI RMF profiles.
Cons of implementing NIST AI RMF:
- Can be resource-intensive, requiring significant time and investment.
- May be complex for organizations new to AI risk management.
- Needs ongoing updates to keep up with evolving AI technologies.
- Initial costs and potential restrictions on innovation may be a concern.
How Scrut can help
Scrut’s automation capabilities help organizations efficiently implement the NIST AI RMF, ensuring responsible AI development and compliance with ethical and legal standards.
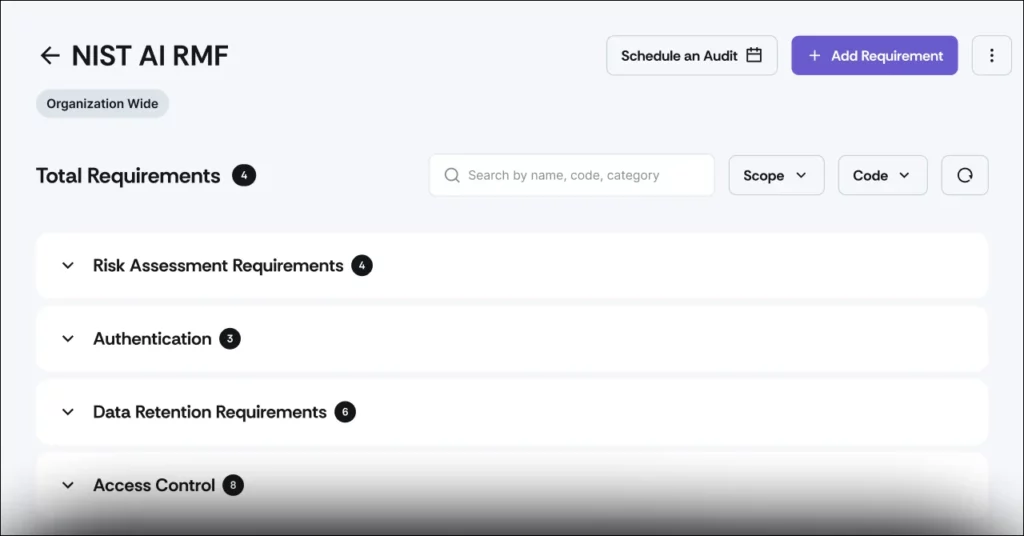
The platform also supports the Govern function, enabling organizations to manage AI technologies securely and responsibly by leveraging continuous control monitoring to track compliance in real time.
Additionally, Scrut enhances AI security training by aligning policies and controls with industry best practices, ensuring robust security and governance for AI technologies.
Let Scrut streamline your compliance efforts and make managing AI risks easier, faster, and more effective. Contact us to learn more.

Grace Arundhati is a passionate writer who specializes in creating engaging and informative pieces on information security, compliance, risk management, and a range of other topics. Outside of writing, Grace enjoys pet parenting, reading, and binge-watching period dramas.