Think AI, and you are sure to come up with thoughts about innovation at the cost of security risks. Though AI does pose risks to information security, it can also be used to enhance Governance Risk management, and Compliance (GRC).
AI use cases in GRC are revolutionizing traditional approaches, ushering in a new era of efficiency, accuracy, and proactive risk management. From automating compliance processes to providing real-time insights for risk mitigation, AI is reshaping how organizations navigate the complex landscape of GRC.
The integration of AI into GRC not only enhances the speed and precision of decision-making but also empowers businesses to stay ahead of evolving regulatory requirements, ensuring a resilient and adaptive framework for sustainable success.
This blog explores how AI can boost governance, risk management, and compliance, and provides some best practices for using AI in GRC.
AI use cases in governance
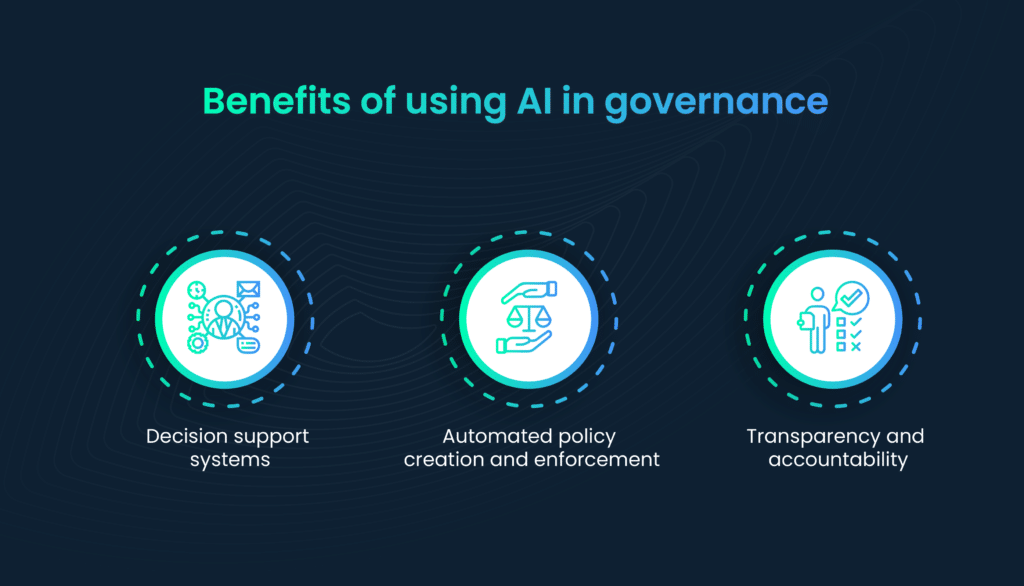
AI plays a transformative role in governance by offering innovative solutions that enhance decision-making processes, streamline policy management, and foster transparency and accountability.
Here’s a breakdown of AI’s key contributions to governance:
A. Integration of AI in decision support systems
AI is transforming decision-making processes within governance structures through its seamless integration into decision support systems.
By leveraging advanced algorithms and data analytics, AI enhances the quality and speed of decision-making.
It provides decision-makers with real-time insights, predictive analytics, and scenario analyses, enabling them to make informed choices that align with organizational goals and regulatory compliance.
The integration of AI not only streamlines decision-making but also contributes to more agile and responsive governance frameworks.
In the financial sector, major investment firms are employing AI-driven decision support systems to optimize portfolio management.
These systems analyze vast datasets, market trends, and historical performance to provide investment managers with real-time insights.
By considering diverse factors, including economic indicators and geopolitical events, AI aids in making informed decisions, mitigating risks, and maximizing returns.
B. Automated policy creation and enforcement with AI
In the realm of governance, AI is reshaping how policies are formulated and enforced. Automated policy creation, powered by AI, involves the efficient analysis of vast datasets to identify regulatory requirements and organizational needs.
AI algorithms can draft, refine, and update policies autonomously, ensuring they align with the latest compliance standards. Furthermore, AI-driven enforcement mechanisms automate the monitoring and implementation of policies, reducing the risk of human error and enhancing overall compliance.
This not only accelerates policy lifecycle management but also fortifies organizations against potential governance pitfalls.
In healthcare compliance, organizations are leveraging AI to streamline the creation and enforcement of privacy policies. AI algorithms analyze evolving healthcare regulations and dynamically update privacy policies to align with compliance standards.
Automated enforcement tools continuously monitor data access, usage, and sharing, ensuring that healthcare providers adhere to strict privacy regulations such as HIPAA. This not only reduces the administrative burden but also enhances patient data security.
C. Enhancing transparency and accountability through AI
AI catalyzes fostering transparency and accountability within governance structures. By implementing AI-powered tools, organizations can gain comprehensive insights into their operations, enabling a clear and traceable audit trail.
AI algorithms monitor and analyze transactions, activities, and decision processes, ensuring adherence to established protocols and regulations. This not only minimizes the risk of non-compliance but also instills a culture of accountability at all levels of the organization.
Through the lens of AI, governance becomes a proactive and transparent endeavor, reinforcing trust among stakeholders and regulatory bodies alike.
Government agencies are incorporating AI to enhance transparency and accountability in public procurement processes. AI-powered systems monitor procurement transactions, flagging any anomalies or deviations from established protocols.
This ensures fair and transparent procurement practices, minimizes the risk of corruption, and instills public trust in government processes.
AI’s ability to provide an auditable trail of procurement activities contributes to accountability and compliance with regulatory standards.
AI use cases in risk management
AI plays a crucial role in risk management within GRC frameworks by offering advanced tools and capabilities to identify, assess, and mitigate risks effectively.
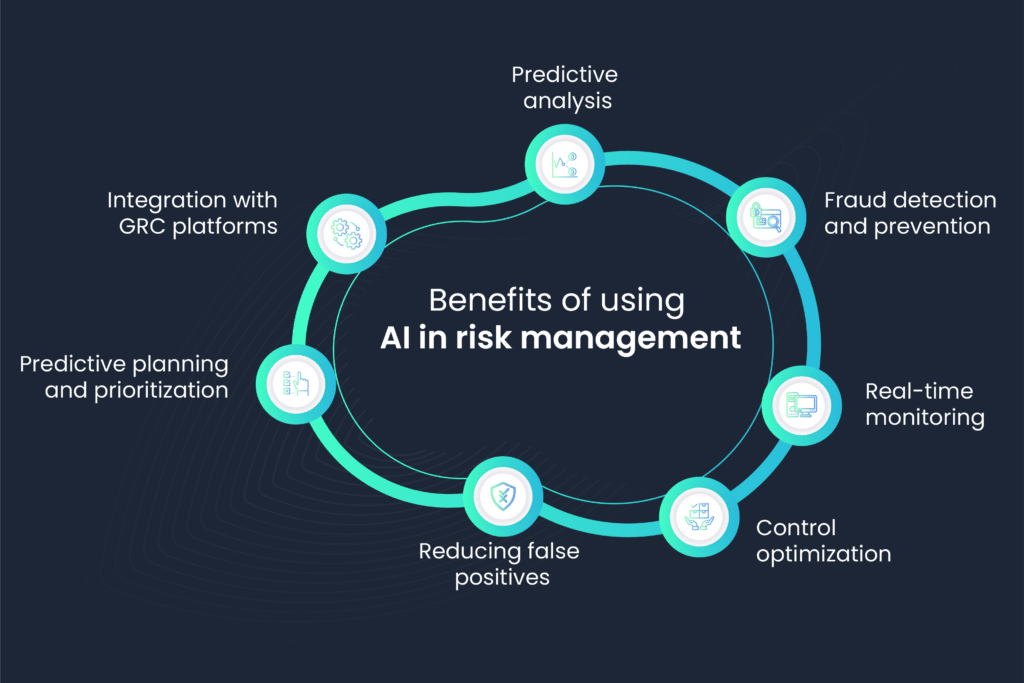
Here are key aspects of AI’s role in risk management:
A. Predictive analytics
AI leverages predictive analytics to forecast potential risks by analyzing historical data, market trends, and external factors. By identifying patterns and correlations, AI can provide organizations with early warnings, enabling proactive risk management strategies.
Financial institutions utilize AI-driven predictive analytics to assess credit risks. The system analyzes customer behavior, financial transactions, and external economic indicators to predict potential defaults.
This proactive approach allows institutions to implement risk mitigation measures and optimize lending strategies.
B. Fraud detection and prevention
In the financial sector and beyond, AI is instrumental in detecting and preventing fraud. Machine learning algorithms can analyze transaction patterns, identify anomalies, and flag potentially fraudulent activities in real-time, reducing financial risks and safeguarding assets.
An e-commerce website may employ AI algorithms to detect fraudulent transactions in real-time. By analyzing patterns of user behavior, and transaction history, and identifying anomalies, the system can automatically flag and prevent potentially fraudulent activities. This minimizes financial losses and safeguards the integrity of the online marketplace.
C. Real-time monitoring
AI enables real-time monitoring of diverse data sources, including financial transactions, cybersecurity events, and operational activities. This continuous monitoring allows organizations to identify and respond swiftly to emerging risks, minimizing the impact on business operations.
Cybersecurity firms utilize AI for real-time monitoring of network activities. AI algorithms continuously analyze network traffic, identify unusual patterns, and detect potential cyber threats.
This enables immediate response to mitigate risks, prevent data breaches and ensure the security of sensitive information.
D. Control optimization
AI assists in optimizing internal controls by evaluating their effectiveness through data analysis. By identifying patterns of over-testing or under-testing controls, AI helps organizations refine and strengthen their control frameworks, ensuring a more robust risk management infrastructure.
Manufacturing companies can integrate AI into their GRC platform to optimize internal controls. The AI algorithms can assess the effectiveness of existing controls by analyzing historical data on production processes. By identifying areas of improvement and potential weaknesses, the companies can enhance their control framework, reducing operational risks.
E. Reducing false positives
In Anti-Money Laundering (AML) and Know Your Customer (KYC) processes, AI contributes to risk management by reducing false positives. Advanced algorithms can sift through vast amounts of data, improving accuracy in identifying suspicious activities and potential compliance risks.
F. Predictive planning and prioritization
AI aids in the prioritization of risk assessments by predicting and assessing the likelihood and impact of various risks. This enables organizations to allocate resources more effectively, focusing on high-priority risks and optimizing risk management strategies.
Logistics companies can utilize AI for predictive planning in their supply chain. AI algorithms can analyze historical data on logistics operations, assess potential risks such as delays or disruptions, and provide insights for proactive planning. This minimizes disruptions, enhances supply chain resilience, and optimizes resource allocation.
G. Integration with GRC platforms
AI is integrated into GRC platforms to enhance their capabilities. GRC software, empowered by AI, can quickly identify and harmonize risk and control libraries, locate missing relationships between risks, controls, and processes, and proactively identify issue trends, emerging risks, and control failures.
Healthcare organizations can integrate AI into their GRC software to enhance risk management. AI can continuously monitor regulatory changes, assess compliance risks, and suggest updates to policies and procedures. This ensures ongoing compliance with evolving healthcare regulations and minimizes legal risks.
AI use cases in compliance
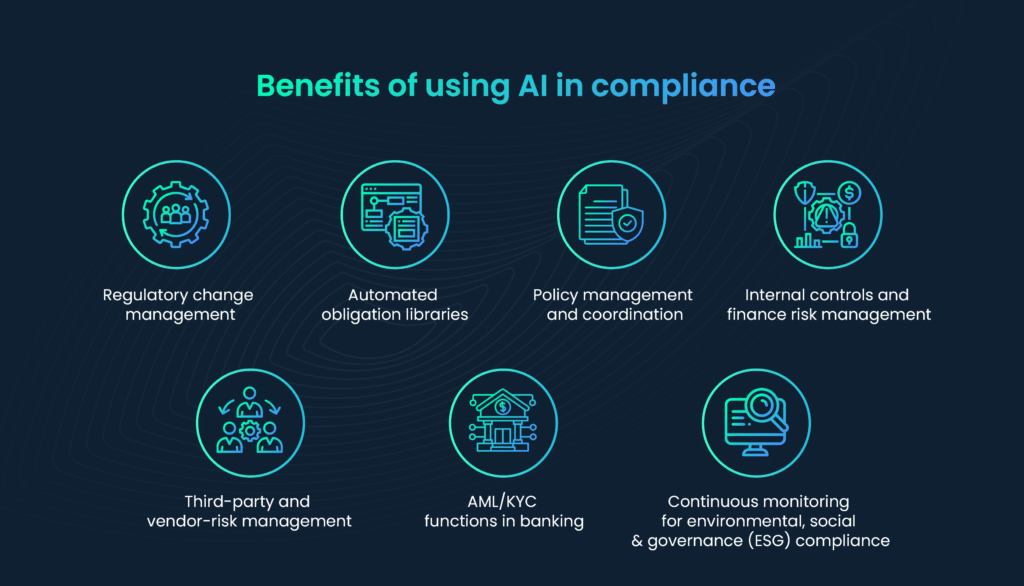
Artificial Intelligence (AI) plays a pivotal role in compliance within GRC frameworks by introducing automation, efficiency, and precision into various compliance-related processes. Here are key aspects of AI’s role in compliance in GRC:
A. Regulatory change management
AI facilitates proactive regulatory change management by continuously monitoring and analyzing an extensive range of sources, including legal databases, government announcements, and industry publications.
Through natural language processing (NLP) and machine learning, AI can interpret complex regulatory language, identify updates, and provide organizations with real-time insights into changes that may impact compliance.
This ensures that organizations stay abreast of evolving regulations and can swiftly adapt their policies and procedures to maintain compliance.
Financial institutions can use AI to monitor and analyze regulatory changes in real-time. AI algorithms can scan legal databases, government announcements, and industry publications to identify updates to financial regulations.
This ensures that organizations remain constantly informed about changes, facilitating prompt adjustments to policies and procedures to maintain compliance.
B. Automated obligation libraries
AI automates the creation and management of obligation libraries by systematically analyzing and cataloging regulatory obligations.
Machine learning algorithms can identify and categorize specific obligations relevant to an organization based on changes in regulations and industry standards.
This automation not only ensures a comprehensive and up-to-date obligation library but also reduces the manual effort required for maintaining compliance records.
In the healthcare sector, AI is employed to automate the creation and management of obligation libraries. The system analyzes healthcare regulations, updates, and industry standards to ensure that the organization’s obligations are accurately cataloged.
This automated process reduces manual effort, minimizes the risk of oversight, and ensures comprehensive compliance.
C. Policy management and coordination
AI enhances policy management by mapping regulations to an organization’s existing policies and procedures. By identifying gaps and suggesting updates, AI ensures that policies consistently align with the evolving compliance landscape.
Additionally, AI-powered coordination ensures that changes in regulations are seamlessly integrated into policy frameworks, reducing the risk of non-compliance due to outdated or misaligned policies.
Enterprises can utilize AI to enhance policy management by automating the coordination between existing policies and regulatory changes. AI algorithms can map regulations to the policies, detect gaps, and suggest necessary updates.
This not only streamlines policy lifecycle management but also ensures that policies consistently align with evolving compliance requirements.
D. Internal controls and finance risk management
Within internal controls and finance risk management, AI contributes by analyzing financial data, evaluating control effectiveness, and identifying trends. Machine learning algorithms can detect patterns indicative of potential risks, control failures, or duplications.
This enables organizations to optimize their internal controls, identify weaknesses in financial risk management, and enhance compliance with financial regulations.
Manufacturing companies can integrate AI into their GRC platforms for internal controls and finance risk management. AI can analyze financial data, identify trends, and evaluate the effectiveness of internal controls.
By proactively detecting control failures or duplications, organizations can strengthen their finance risk management and ensure compliance with financial regulations.
E. Third-party and vendor-risk management
AI revolutionizes third-party and vendor-risk management by automating risk assessments. Machine learning algorithms assess various factors, including financial stability, cybersecurity practices, and the compliance history of vendors.
This automated risk evaluation streamlines due diligence processes, identifies potential risks associated with external partners, and ensures compliance with regulatory standards in vendor relationships.
In the retail industry, AI is utilized to assess and monitor third-party and vendor risks. The system employs AI algorithms to evaluate the financial stability, cybersecurity practices, and compliance history of vendors.
This automated risk assessment enhances due diligence processes and ensures that the organization’s partners comply with relevant regulations.
F. AML/KYC functions in banking
In Anti-Money Laundering (AML) and Know Your Customer (KYC) functions, AI provides advanced capabilities for analyzing vast volumes of customer data. Machine learning algorithms detect patterns indicative of money laundering, fraudulent activities, or suspicious transactions.
This not only enhances the accuracy of risk assessments but also ensures compliance with stringent financial regulations governing customer identification and transaction monitoring.
Continuous monitoring for environmental, social & governance (ESG) compliance
AI enables continuous monitoring for ESG compliance by analyzing data related to environmental impact, social responsibility, and governance practices. Machine learning algorithms can assess adherence to ESG standards, identify areas for improvement, and ensure ongoing compliance.
This proactive approach allows organizations to demonstrate a commitment to sustainable practices and align with evolving ESG requirements.
Aspect | Governance | Risk Management | Compliance |
AI’s role | Enhances decision-making processes, streamline policy management, and foster transparency and accountability | Offers advanced tools and capabilities to identify, assess, and mitigate risks effectively | Introduces automation, efficiency, and precision into various compliance-related processes |
AI use cases | Decision support systems, automated policy creation and coordination | Predictive analytics for risk forecasting, fraud detection and prevention, real-time monitoring for cybersecurity | Regulatory change management, automated obligation libraries, policy management and coordination |
Best practices for using AI in GRC
Finding a GRC solution with AI is a great idea for your organization, but it requires careful consideration and adherence to best practices. Here are some key guidelines for the effective use of AI in GRC:
A. Define clear objectives
Clearly define the objectives and goals of implementing AI in GRC. Whether it’s enhancing decision-making, automating compliance processes, or optimizing risk management, having well-defined objectives helps in selecting the right AI applications and measuring success.
B. Understand the data landscape
Gain a deep understanding of the data landscape within your organization. AI relies on quality data for accurate analysis and insights. Ensure data integrity, accuracy, and accessibility, and implement data governance practices to maintain data quality throughout its lifecycle.
C. Ensure regulatory compliance
Stay abreast of relevant regulations and compliance standards associated with AI applications. Understand the ethical implications of AI, particularly in decision-making processes, and ensure that AI implementations comply with industry regulations and legal requirements.
D. User training and awareness
Provide training to users and stakeholders involved in GRC processes to ensure they understand how AI applications work and how to interpret the insights generated. Building awareness and trust among users is crucial for the successful integration of AI into GRC workflows.
E. Continuous monitoring and evaluation
Implement mechanisms for continuous monitoring and evaluation of AI applications. Regularly assess the performance of AI models, monitor for biases, and validate the accuracy of predictions. This ensures that AI remains effective and aligned with the evolving needs of GRC.
F. Data security and privacy
Prioritize data security and privacy considerations. Implement robust cybersecurity measures to protect sensitive GRC data. Ensure that AI applications adhere to privacy regulations and standards, and consider using techniques like federated learning to train models without centralizing sensitive data.
G. Human oversight
Integrate human oversight into AI processes, especially in critical decision-making scenarios. While AI can automate and optimize many tasks, human judgment is essential to validate results, interpret complex situations, and handle exceptions that may not be covered by AI algorithms.
H. Interdisciplinary collaboration
Foster collaboration between IT, data science, legal, compliance, and business teams. Establish interdisciplinary teams to ensure a holistic approach to AI in GRC. This collaboration helps align AI initiatives with organizational goals, compliance requirements, and risk management strategies.
I. Transparency and explainability
Prioritize transparency and explainability in AI models. Ensure that AI-driven decisions are interpretable and understandable by stakeholders. Transparent AI models build trust among users and regulators, which is essential in compliance and risk management contexts.
J. Regular audits and assessments
Conduct regular audits and assessments of AI systems. This includes reviewing the algorithms, data inputs, and outputs to identify and rectify any biases, inaccuracies, or issues that may arise over time. Regular assessments ensure ongoing compliance and reliability of AI applications.
Wrapping up
While the mention of AI often evokes concerns about security risks, its integration into GRC has proven to be a transformative force. AI use cases in GRC are reshaping traditional approaches, ushering in an era of efficiency, accuracy, and proactive risk management.
From automating compliance processes to providing real-time insights for risk mitigation, AI is fundamentally altering how organizations navigate the intricate landscape of GRC.
The integration of AI not only enhances the speed and precision of decision-making but also empowers businesses to stay ahead of evolving regulatory requirements, ensuring a resilient and adaptive framework for sustainable success.
With a careful and strategic approach, businesses can leverage AI to enhance their GRC strategies, fortify compliance efforts, and proactively manage risks in an ever-evolving landscape.
Explore how AI can boost your GRC program by scheduling a demo with us today!
FAQs
AI plays a multifaceted role in GRC, enhancing decision-making, automating compliance processes, optimizing risk management, and fostering transparency and accountability. It introduces innovative solutions to streamline traditional approaches in the business landscape.
AI seamlessly integrates into decision support systems, leveraging advanced algorithms and data analytics to provide real-time insights, predictive analytics, and scenario analyses. This empowers decision-makers to make informed choices aligned with organizational goals and regulatory compliance, contributing to more agile and responsive governance frameworks.
AI serves as a catalyst for transparency and accountability by providing comprehensive insights into operations, creating a clear and traceable audit trail. AI algorithms monitor transactions, activities, and decision processes, ensuring adherence to established protocols and regulations. This minimizes the risk of non-compliance and fosters a culture of accountability at all levels of the organization.
AI plays a crucial role in risk management by offering advanced tools and capabilities for identifying, assessing, and mitigating risks effectively. It leverages predictive analytics, fraud detection, real-time monitoring, control optimization, and reduction of false positives to enhance risk management strategies.
AI plays a pivotal role in compliance by introducing automation, efficiency, and precision into various compliance-related processes. It facilitates regulatory change management, automates obligation libraries, enhances policy management, and optimizes internal controls and finance risk management.